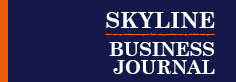
PRINT ISSN 1998-3425
Jesús Constant, Business School, Tecnologico de Monterrey, Mexico
Jaime Ruiz, Business School, Tecnologico de Monterrey, Mexico
Martha Martínez, Business School, Tecnologico de Monterrey, Mexico
Orlando López, Business School, Tecnologico de Monterrey, Mexico
Jesús C. Téllez, Business School, Tecnologico de Monterrey, Mexico
The U.S. unemployment rate serves as a critical economic indicator, playing a pivotal role in assessing economic well-being and societal welfare and attracting the attention of both national and international corporations. The present study takes into account the underlying causes, effects, and potential remedies associated with the unemployment rate. The study also aims to construct a robust forecasting methodology for the unemployment rate indicator, with the help of diverse set of variables such as the S&P 500 index, inflation rates, currency fluctuations, and other associated factors. Several statistical models, including logistic regression OLS (Ordinary Least Square), neural networks, k-nearest neighbours, and supervised machine learning algorithms, have been examined. In the present study, the logistic regression model achieved a relatively high accuracy of 67%, which is compatible with the inherent volatility of the unemployment rate.
Constant J., Ruiz, J., Martínez, M., López,, O., & Téllez, J. C. (2024). Forecasting the Unemployment Rate in the United States. Skyline Business Journal, 20(1), 25-33.